Mathematical models enable a scientific understanding of natural phenomena around us, and the study and optimization of processes in society and industry.
Research activity
We fuse classical physics-based modelling and new machine learning methods for the identification and training of entirely new models based on few observations and sparse data.
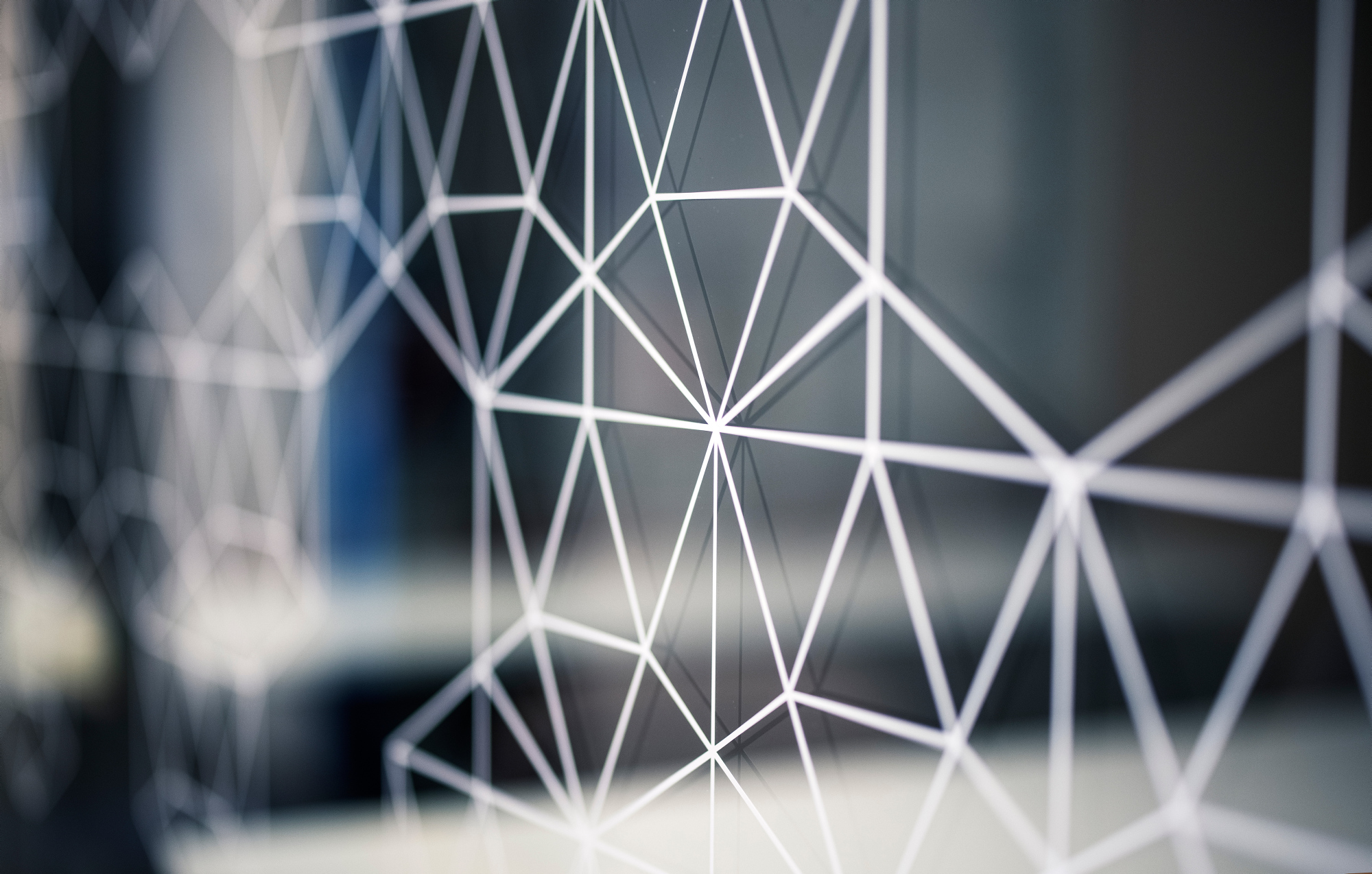
Advances in computing technology, in machine learning approaches and the availability of enormous data sets have resulted in highly successful “trained machines” with their own underlying neural network structures generated only from observations. Such algorithms can be highly effective within its own comfort zone, i.e., for operating and making predictions within the boundary of its training data set. However, such approaches normally require vast amount of data to become reliable and accurate and consume considerable power in the process. Furthermore, such approaches tend to lose their predictive capabilities when faced with previously unseen scenarios, and they provide very limited analytical insights into the fundamental mechanisms that are at play – these are essential for the modeler.
An alternative approach that can remedy these shortcomings, is to fuse modern machine learning methods with fundamental physical rules, such as conservation principles for mass, momentum and energy, and well-defined boundary conditions that the training architecture must respect. This could be in the form of defining general partial differential equations (PDEs) that are rooted in the physics, and that govern how our primary variables evolve in space and time, and then turn this into a learning tool by populating it with a priori unknown model parameters. This physically sound “learning machine” can now be trained with a small volume of data and carries predictive capability even for unseen scenarios after training.
We perform fundamental and applied research within modelling of problems that have both societal and industrial relevance. We explore different types of PDE models using classical techniques, but increasingly also neural network structures and machine learning based techniques as well as Bayesian methods like ensemble Kalman filter methods. We refer to the publication list for different concrete examples and application areas (click the picture to get access to the publication data). Recent works focus on systems in the context of fluid mechanics in a broad sense.
Examples of such applications we currently have focus on are:
- General conservation laws
- Wellbore related operations like displacement of fluids and gas leakage
- Digital tumor based on mathematical multiphase models
- Battery system
Ongoing PhD projects
- Maryam Ghorbani: Experimental and computational study of density-unstable reverse-circulation displacements/Eksperimentell og numerisk studie av tetthetsustabil fortrengning for sementering ved reverssirkulering.
- Yao Zhang: Experiments and modelling of immiscible displacements and removal of wall films/Eksperimenter og modellering av fortrengning med ikke-blandbare væsker og fjerning av tynne væskefilmer.
- Åsmund Aamodt Resell: Computational study of annular well flow and drill string vibration/Beregningsbasert studenter av brønnstrømning og borestrengvibrasjon
Recent PhD projects
- Qing Li (2023): Identification of Nonlinear Conservation Laws using Symbolic Neural Networks.
- Jahn Otto Waldeland (2020): Mathematical modeling of cancer cell migration - from in vitro to in vivo.
- Yangyang Qiao (2020): Mathematical modeling of compressible and incompressible multiphase flow based on mixture theory.
People
Department of Energy and Petroleum Engineering
Department of Energy and Petroleum Engineering